In the age of information explosion, the digital world is generating data at an unprecedented rate. This surge has catalyzed the emergence of fields dedicated to extracting insights and value from this deluge of data. Two such disciplines at the forefront are Data Analytics and Data Science. While often used interchangeably, these terms encapsulate distinct methodologies, skill sets, and objectives. In this article, we embark on a journey to decipher the fine line between Data Analytics and Data Science, exploring their definitions, methodologies, applications, and the pivotal role each plays in today’s data-driven landscape.
Defining Data Analytics and Data Science:
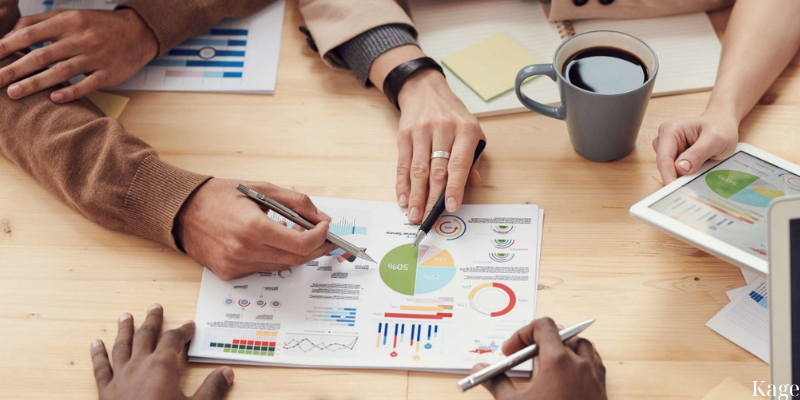
Data Analytics and Data Science are intertwined disciplines, yet their scopes and methodologies diverge significantly.
Data Analytics primarily involves the exploration, cleansing, and interpretation of structured data to extract actionable insights and inform decision-making processes. It encompasses a broad spectrum of techniques, including descriptive statistics, data mining, and visualization, aimed at uncovering patterns, trends, and correlations within datasets. The key emphasis of data analytics lies in answering specific business questions, optimizing processes, and facilitating data-driven decision-making.
On the other hand, Data Science is a multifaceted field that encompasses a broader range of activities, including data collection, preprocessing, analysis, and predictive modeling, often leveraging advanced algorithms and machine learning techniques. Data Science delves deeper into the data lifecycle, focusing not only on extracting insights but also on predicting future trends, generating actionable recommendations, and building data-driven products or systems.
Methodologies and Techniques:
Data Analytics predominantly relies on descriptive and diagnostic analytics techniques to summarize historical data, identify patterns, and diagnose problems. Descriptive analytics involves summarizing and visualizing data to gain insights into past trends and performance. Diagnostic analytics delves deeper into understanding why certain events occurred by analyzing historical data and identifying root causes.
In contrast, Data Science encompasses a more extensive array of methodologies, including predictive and prescriptive analytics. Predictive analytics involves forecasting future trends and outcomes based on historical data patterns, employing techniques such as regression analysis, time series analysis, and machine learning algorithms. Prescriptive analytics takes predictive insights a step further by recommending optimal actions to achieve specific objectives, often through the application of optimization and simulation techniques.
Applications and Impact:
Both Data Analytics and Data Science find applications across various industries and domains, driving innovation, efficiency, and informed decision-making.
Data Analytics is widely employed in business intelligence, marketing analytics, customer segmentation, and operational optimization. Businesses use analytics to gain insights into consumer behavior, improve marketing strategies, optimize supply chain operations, and enhance overall performance and competitiveness.
Data Science, with its predictive and prescriptive capabilities, powers a myriad of applications ranging from recommender systems and predictive maintenance to fraud detection and personalized healthcare. In e-commerce, Data Science algorithms analyze user behavior to offer personalized product recommendations, enhancing user experience and driving sales. In healthcare, predictive analytics models leverage patient data to forecast disease outbreaks, optimize treatment protocols, and improve healthcare delivery.
The Evolution of Data Analytics and Data Science:
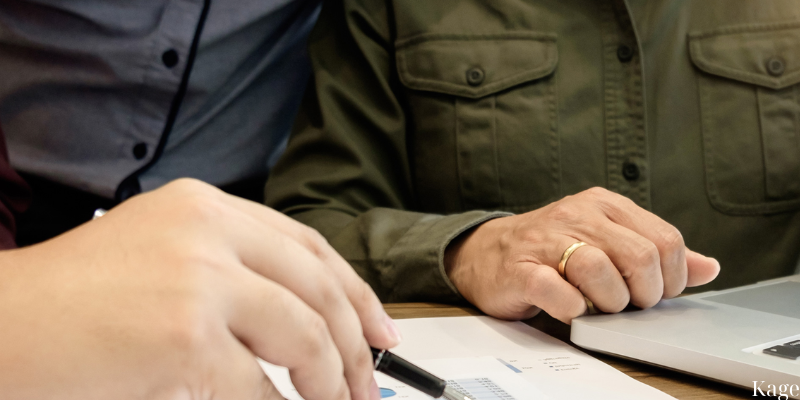
The fields of Data Analytics and Data Science have witnessed rapid evolution fueled by advancements in technology, the proliferation of big data, and the growing demand for data-driven insights.
Data Analytics has evolved from traditional business intelligence tools to sophisticated analytics platforms capable of processing vast volumes of data in real-time. The integration of artificial intelligence (AI) and machine learning algorithms has empowered data analysts to uncover deeper insights and make more accurate predictions, thereby enhancing decision-making capabilities.
Similarly, Data Science has transformed, driven by innovations in machine learning, deep learning, and artificial intelligence. The advent of big data technologies, such as Hadoop and Spark, has enabled data scientists to tackle complex problems and extract valuable insights from massive datasets. Moreover, the democratization of machine learning frameworks and libraries has lowered the barrier to entry, allowing individuals and organizations to leverage advanced analytics capabilities.
Challenges and Future Trends:
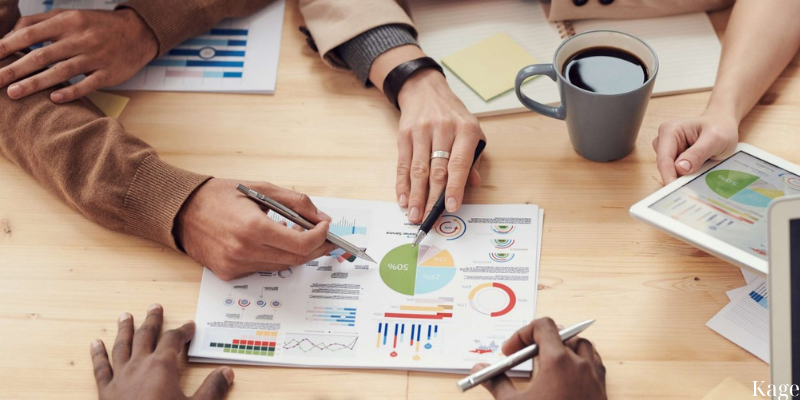
While Data Analytics and Data Science hold immense promise, they also face challenges and opportunities on the horizon.
Data Analytics faces challenges related to data quality, privacy concerns, and the need for interdisciplinary collaboration. As organizations grapple with ever-increasing volumes of data, ensuring data accuracy, relevance, and integrity remains paramount. Moreover, with the enactment of stringent data privacy regulations such as GDPR and CCPA, organizations must navigate the complex landscape of data governance and compliance.
Data Science confronts challenges associated with algorithm bias, interpretability, and scalability. Machine learning algorithms are susceptible to bias inherent in training data, raising ethical concerns surrounding algorithmic fairness and transparency. Moreover, as the scale and complexity of datasets continue to grow, data scientists must explore scalable solutions and innovative techniques to extract meaningful insights and ensure model robustness.
Looking ahead, the convergence of Data Analytics and Data Science is poised to drive transformative change across industries, unlocking new opportunities for innovation and growth. With advancements in AI, machine learning, and big data technologies, organizations can harness the power of data to gain a competitive edge, drive operational efficiencies, and deliver value to customers.
Conclusion:
In conclusion, Data Analytics and Data Science represent two sides of the same coin, each playing a crucial role in extracting insights and value from data. While Data Analytics focuses on descriptive and diagnostic analytics to uncover patterns and inform decision-making, Data Science extends beyond, leveraging predictive and prescriptive analytics to forecast future trends and recommend optimal actions. As organizations navigate the complexities of the data-driven landscape, understanding the nuances between Data Analytics and Data Science is essential for harnessing the full potential of data to drive innovation, efficiency, and growth.