In today’s era of digital innovation, data analytics has emerged as a transformative tool in the realm of healthcare. Specifically, population health data analytics has garnered significant attention for its potential to revolutionize healthcare delivery, improve patient outcomes, and optimize resource allocation. This article delves into the intricate landscape of population health data analytics, exploring its evolution, applications, challenges, and the promising future it holds in shaping a healthier world.
Evolution of Population Health Data Analytics:
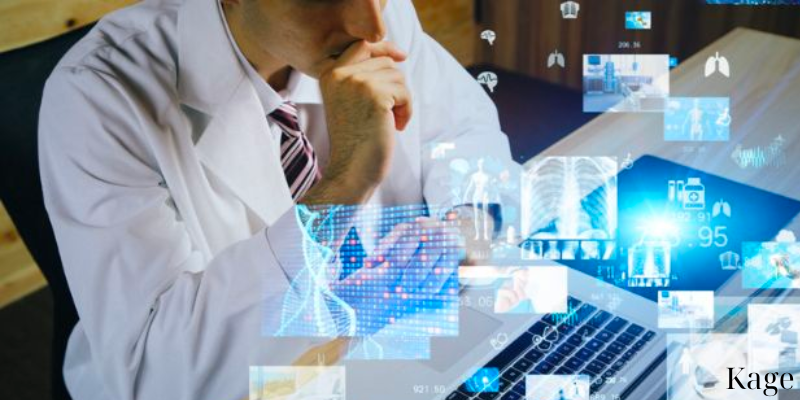
The journey of population health data analytics traces back to the early 2000s when advancements in technology began to converge with the growing need for efficient healthcare management. At its nascent stage, healthcare data primarily comprised electronic health records (EHRs) and claims data, providing limited insights into population health trends. However, with the advent of big data analytics and machine learning algorithms, healthcare stakeholders gained the capability to analyze vast volumes of structured and unstructured data from diverse sources.
Applications of Population Health Data Analytics:
The applications of population health data analytics are multifaceted and extend across various domains within healthcare. One of the primary uses is disease surveillance, where analytics tools can track the prevalence of diseases, identify outbreaks, and facilitate proactive interventions. Moreover, predictive analytics plays a pivotal role in forecasting disease patterns, enabling healthcare providers to allocate resources efficiently and implement preventive measures.
Furthermore, population health data analytics is instrumental in enhancing care coordination and management. By integrating data from disparate sources, such as EHRs, wearable devices, and social determinants of health, healthcare organizations can gain a comprehensive understanding of patients’ health status and risk factors. This enables personalized care interventions, proactive outreach, and targeted interventions to improve health outcomes.
Challenges in Population Health Data Analytics:
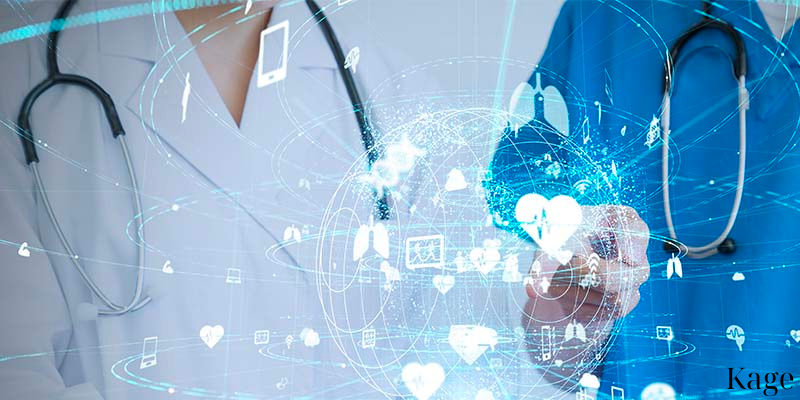
Despite its immense potential, population health data analytics faces several challenges that hinder its widespread adoption and effectiveness. One of the foremost challenges is data interoperability and integration. Healthcare data resides in siloed systems, making it difficult to aggregate and analyze information seamlessly. Additionally, ensuring data accuracy, privacy, and security poses significant concerns, especially amidst the growing threat of data breaches and cyberattacks.
Another critical challenge is the lack of standardized metrics and methodologies for population health analysis. The absence of uniformity makes it challenging to compare outcomes across different populations and evaluate the efficacy of interventions accurately. Moreover, there is a shortage of skilled data analysts and data scientists proficient in healthcare analytics, exacerbating the gap between data generation and actionable insights.
Future Directions and Opportunities:
Despite the challenges, the future of population health data analytics appears promising, driven by technological advancements, policy initiatives, and evolving healthcare models. The emergence of interoperability standards, such as HL7 FHIR and SMART on FHIR, holds the potential to facilitate seamless data exchange and interoperability across healthcare systems.
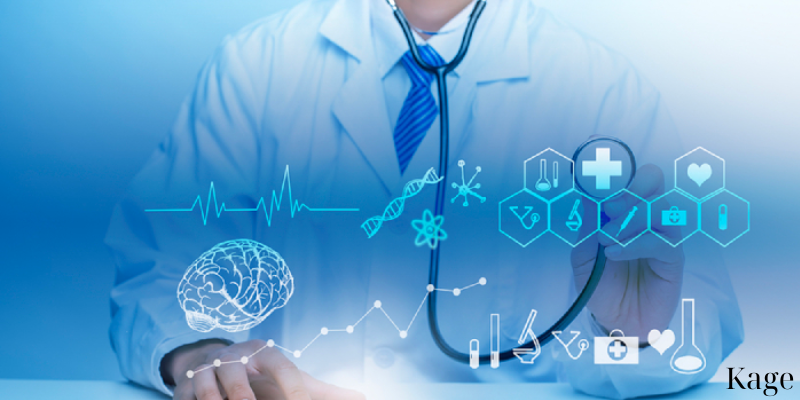
Moreover, advancements in artificial intelligence (AI) and machine learning (ML) are poised to unlock new frontiers in population health analytics. These technologies can leverage complex algorithms to uncover hidden patterns, predict disease trajectories, and generate actionable insights in real-time. Additionally, the proliferation of remote patient monitoring devices and digital health platforms enables continuous data collection and monitoring, empowering individuals to actively participate in managing their health.
Furthermore, the shift towards value-based care models and population health management initiatives incentivizes healthcare organizations to invest in data analytics capabilities. By leveraging data-driven insights to drive preventive care, reduce hospital readmissions, and improve care coordination, healthcare providers can achieve better outcomes at lower costs.
Conclusion:
Population health data analytics represents a paradigm shift in healthcare delivery, offering unprecedented opportunities to improve population health outcomes and enhance the efficiency of healthcare systems. Despite facing challenges related to data interoperability, privacy, and skill shortages, the continued advancement of technology and concerted efforts from healthcare stakeholders hold the promise of overcoming these obstacles.
As we navigate towards a future characterized by data-driven healthcare, embracing population health data analytics is imperative to unlock its full potential in shaping a healthier and more equitable world. By harnessing the power of data analytics, we can drive meaningful change, optimize resource allocation, and ultimately, improve the well-being of individuals and communities worldwide.